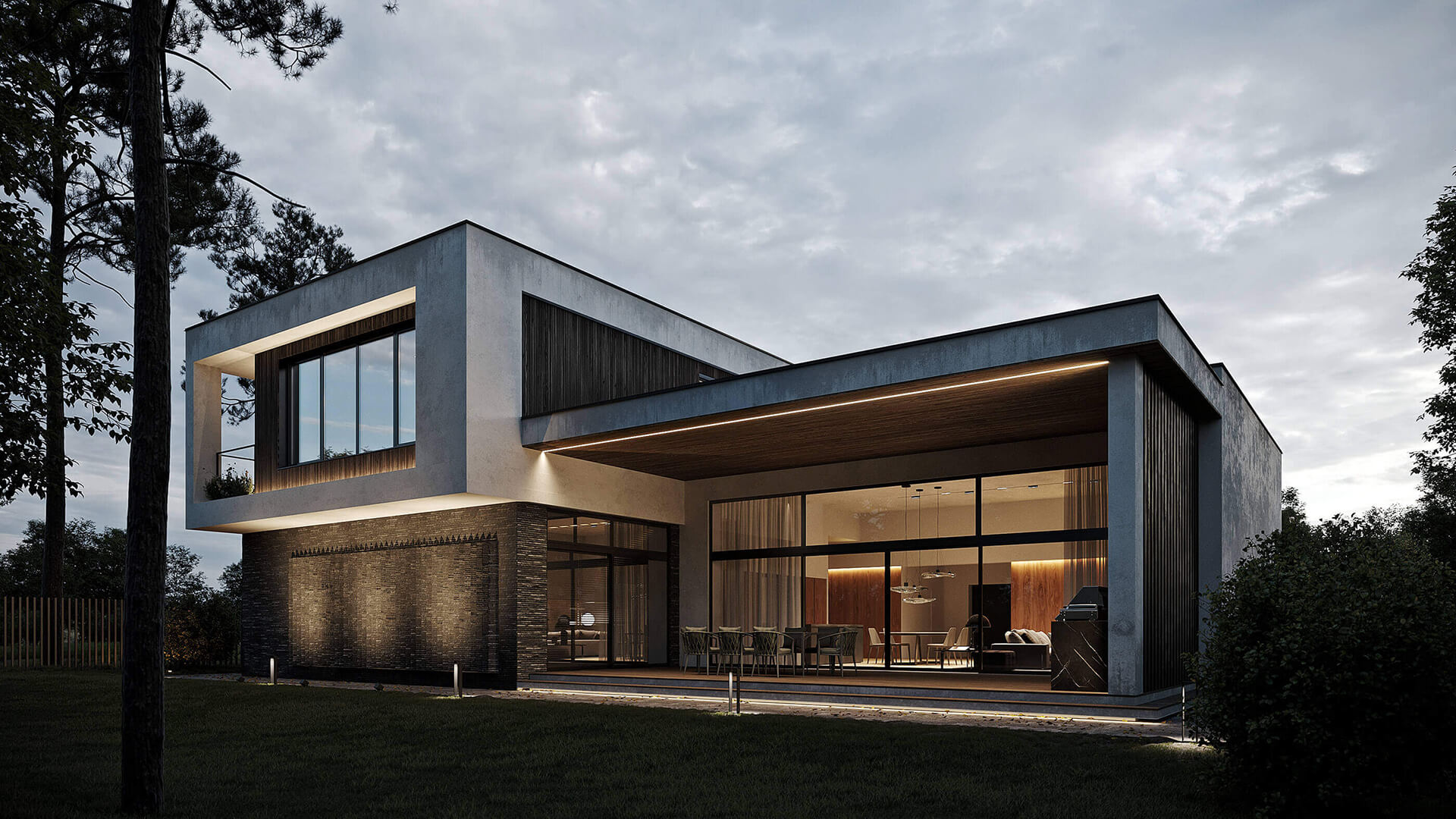
Into better of our knowledge, our company is the first ever to conduct a methodical study on the location confidentiality leakage possibilities caused by the vulnerable interaction, also app style flaws, of established typical proximity-based programs.
(i) Track place Ideas streams and Evaluating the Risk of Location Privacy leaks in prominent Proximity-Based applications. Also, we investigate an RS application named Didi, the greatest ridesharing app that contains taken over Uber Asia at $35 billion bucks in 2016 and from now on acts significantly more than 300 million unique passengers in 343 locations in Asia. The adversary, for the capability of a driver, can accumulate several trips requests (for example., consumer ID, departure times, deviation spot, and resort put) of nearby passengers. Our very own study shows the broader existence of LLSA against proximity-based apps.
(ii) Proposing Three standard assault Methods for place Probing and studying Them via various Proximity-Based programs. We suggest three general fight techniques to probe and track customers’ area suggestions, that can easily be placed on almost all of established NS software. We furthermore discuss the scenarios for using various attack strategies and display these processes on Wechat, Tinder, MeetMe, Weibo, and Mitalk independently. These fight techniques may also be typically relevant to Didi.
(iii) Real-World assault screening against an NS application and an RS application. Thinking about the confidentiality sensitiveness regarding the individual trips info, we found real-world assaults testing against Weibo and Didi therefore to get a great deal of areas and ridesharing demands in Beijing, China. In addition, we execute detailed investigations from the obtained data to show that adversary may obtain ideas that enhance consumer confidentiality inference through the information.
(iv) security Evaluation and Recommendation of Countermeasures. We evaluate the practical defense strength against LLSA of popular apps under investigation. The results suggest that existing defense strength against LLSA is far from sufficient, making LLSA feasible and of low-cost for the adversary. Therefore, existing defense strength against LLSA needs to be further enhanced. We suggest countermeasures against these privacy leakage threats for proximity-based apps. In particular, from the perspective of the app operator who owns all users request data, we apply the anomaly-based method to detect LLSA against an NS app (i.e., Weibo). Despite its simplicity, the method is desired as a line-of-defense of LLSA and can raise the bar for performing LLSA.
Roadmap. Point 2 overviews proximity-based software. Area 3 information three basic attack methods. Part 4 carries out extensive real-world approach evaluating against an NS app named Weibo. Point 5 implies that these problems will also be applicable to popular RS software known as Didi. We measure the protection strength of popular proximity-bases applications and indicates countermeasures ideas in part 6. We present relevant operate in part 7 and deduce in point 8.
Today, thousands of people are utilizing numerous location-based myspace and facebook (LBSN) apps to fairly share interesting location-embedded suggestions with others in their social media sites, while concurrently growing their own internet sites making use of the latest interdependency derived from their locations . The majority of LBSN programs is roughly split into two groups (we and II). LBSN applications of classification we (i.e., check-in apps) motivate customers to generally share location-embedded details using their friends sito incontri avventura, such as for example Foursquare and Bing+ . LBSN software of category II (for example., NS software) focus on myspace and facebook knowledge. Such LBSN apps enable people to locate and connect with strangers around centered on their unique area proximity and then make brand new buddies. In this paper, we give attention to LBSN software of classification II since they match the trait of proximity-based software.